Intensified Competition in Large Models: Alibaba Cloud Boosts AI Infrastructure
The subsequent investment in large models requires greater expenditure on computing power, lower model prices, and higher technical barriers.
This means that the elimination round has already begun, and the competition for large models is intensifying.
Before 2023, a data center with 10,000 AI (Artificial Intelligence) chips was the entry ticket for foundational large models.
After 2024, there is a trend for foundational large models to evolve towards a base of 100,000 AI chips.
Against this backdrop, tech companies with cloud computing businesses, such as Microsoft, Amazon, Google, Alibaba, etc., are increasing their investment efforts.
Large models are "money-eating beasts."
At the hardware level, they require substantial capital expenditures for purchasing chips and servers, and leasing land to build data centers.
At the software level, they need to continuously consume computing power for model training and iteration.
This directly results in a significant increase in the capital expenditure growth rate of these companies like Microsoft, Amazon, Google, and Alibaba.
In the first half of 2024, the total capital expenditure of Microsoft, Amazon, and Google reached $48.6 billion, a year-on-year increase of 75%, reaching the highest peak since 2019.
Advertisement
Alibaba Group's financial report shows that Alibaba's capital expenditure in the first half of 2024 was 23.24 billion yuan, a year-on-year increase of 123.2%.
Alibaba's capital expenditure growth rate in the first half of 2024 also reached its peak since 2019. International market research firm Gartner data shows that Alibaba Cloud is currently the fourth-largest cloud vendor in the world, with a share of 7.9%, just behind Microsoft, Amazon, and Google.
As a Chinese cloud vendor, its moves in the wave of large models are particularly worth paying attention to.
Why does Alibaba Cloud need to make such a large investment in AI?
How long will Alibaba Cloud's AI infrastructure investment continue?
On September 19, Wu Yongming, CEO of Alibaba Group, Chairman and CEO of Alibaba Cloud Intelligence, expressed several important judgments at the Yunqi Conference.
First, over the past 22 months, the development speed of AI has exceeded other historical periods, and it is still in the early stages of large model transformation.
Large model technology is rapidly iterating, and the usability of technology has greatly improved.
The cost of model inference has decreased exponentially, far exceeding Moore's Law.
Inference cost is the key to the outbreak of applications, and Alibaba Cloud will strive to reduce costs.
The threshold for investment in the competition of advanced models worldwide will reach the level of billions or tens of billions of dollars.
Second, the CPU (Central Processing Unit) dominated computing system is accelerating the shift towards the GPU (Graphics Processing Unit) dominated AI computing system.
More than 50% of the new demand in the additional computing power market is generated by AI, and this trend is still expanding.
All industries need infrastructure that is more powerful, larger in scale, and more adapted to AI needs.
In the past year, Alibaba Cloud has invested in a large number of AI computing power, but it still cannot meet customer needs.
Wu Yongming expressed Alibaba's determination to continue to increase AI computing power investment.
He said frankly that the penetration rate of new technologies in the early stage is relatively low, and most people will instinctively doubt, which is normal.
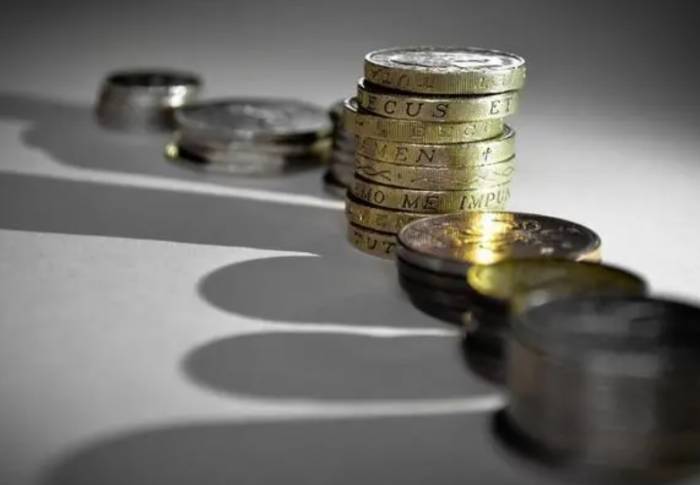
However, new technologies will grow in doubt, and many people will miss it in hesitation.
Alibaba Cloud is making a rare high-intensity investment in AI technology research and development and infrastructure construction.
In Alibaba's financial report conference call for the first quarter of the fiscal year 2025 (i.e., the second quarter of 2024), the Alibaba management disclosed that in the next few quarters, it is expected to continue to maintain a high-speed growth in artificial intelligence capital expenditure.
The development of large models requires continuous investment in AI computing power.
These investments are not just one-time expenditures, but continue for many years.
Because large models need to iterate and upgrade performance, each generation of model parameters and data volume will be larger, requiring more computing power.
In September this year, several cloud vendors' infrastructure technology personnel said to us that ten thousand cards (10,000 AI chips) are just the entry ticket for large models.
Currently, the computing power consumption of the next generation of large models is evolving towards the direction of 100,000 cards, and subsequent computing power investment will only be higher.
Manufacturers that can continue to invest will gradually decrease, and in the end, only a few top manufacturers will continue to participate in the long run.
Taking NVIDIA A100/A800 series AI chips as an example, the price of a single card exceeds 100,000 yuan.
The purchase cost of AI chips for a ten-thousand-card cluster exceeds 1 billion yuan, and the infrastructure cost of a ten-thousand-card smart computing center exceeds 3 billion yuan.
There are very few enterprises that can bear such high costs.
Huge computing power investment has been reflected in the capital expenditure of technology companies.
As the competition for large models intensifies, major technology companies with cloud computing businesses (such as Microsoft, Amazon, Google, etc.)
are increasing their investment in AI computing power.
This has led to a rapid increase in their capital expenditure.
Under normal circumstances, the growth rate of capital expenditure for technology companies is usually around 20%.
However, in the first half of 2024, the capital expenditure of Microsoft, Amazon, and Google was $33 billion, $30.3 billion, and $25.2 billion, respectively, with increases of 78%, 32%, and 91%, respectively.
Microsoft disclosed in the financial report conference call for the fourth quarter of the fiscal year 2024 (i.e., the second quarter of 2024) that the capital expenditure of $19 billion for that quarter was almost entirely used for computing power investment.
The management of Microsoft, Amazon, and Google all stated in the financial report conference call for the second quarter of 2024 that the capital expenditure for the whole year of 2024 will maintain a high-speed growth trend.
Alibaba's computing power investment is also accelerating, and the growth rate is not inferior to international manufacturers.
We have calculated the capital expenditure of Alibaba Group after the first quarter of 2019.
From the first quarter of 2019 to the second quarter of 2024, the average growth rate of Alibaba's capital expenditure per quarter is 15%.
With the acceleration of AI computing power investment, Alibaba's capital expenditure in the first half of 2024 was 23.24 billion yuan, a year-on-year increase of 123.2%.
Among them, the capital expenditure in the first quarter of 2024 was 11.15 billion yuan, with a year-on-year increase of up to 220.4%.
Alibaba's capital expenditure growth rate in the past half year has also reached its peak since 2019.
Alibaba's high-intensity AI computing power investment is beginning to show initial results.
In the second quarter of 2024, Alibaba Cloud's revenue was 26.55 billion yuan, a year-on-year increase of 5.9%.
Alibaba's management disclosed in the post-financial report conference call that Alibaba Cloud's public cloud revenue is maintaining double-digit growth, and AI-related product revenue is maintaining triple-digit growth.
It is expected that Alibaba Cloud's revenue growth rate in the second half of the year will be further improved.
In the competition of large models, the amount of computing power resources is very important, and the efficiency of computing power is even more important.
Large models will consume a lot of computing power during the training stage and inference stage.
The former mainly affects the model manufacturer's model production cost, and the latter affects the cost of enterprise customers using the model.
Zhou Jingren showed the full picture of Alibaba Cloud's AI infrastructure at this Yunqi Conference.
In his view, cloud vendors need to upgrade computing, networking, storage, and other technologies in conjunction to improve computing efficiency.
To improve computing power efficiency, it is necessary to first improve the training efficiency of large models.
An AI computing power cluster is generally composed of thousands of cards and tens of thousands of cards.
The larger the computing power cluster and the more chip quantities, the higher the fault rate will be.
The training of large models is a synchronous task of thousands of cards and tens of thousands of cards.
A failure of a card will affect the operation of the entire cluster.
A cloud vendor's infrastructure technology personnel said to us in September this year that the downtime of AI computing power clusters is directly proportional to the scale of the cluster.
He mentioned a formula-effective AI computing power = single card computing power efficiency × parallel computing efficiency × effective training time.
Each of these items is a multiplicative relationship, and any slight deviation in the performance of any item will have a systemic impact on the overall computing power utilization rate.
Generally, the effective training time of a thousand-card cluster is 99%, but the effective training time of a ten-thousand-card cluster will be reduced to 90%, and the effective training time of a hundred-thousand-card cluster is even close to 0%.
He said frankly that the computing power utilization efficiency of some enterprises is very low.
Some enterprises, when training large models, have a computing power utilization rate of less than 50%.
A large amount of expensive and rare AI computing power is wasted.
Alibaba Cloud CTO (Chief Technology Officer) Zhou Jingren announced at the Yunqi Conference on September 19 that Alibaba Cloud's ten-thousand-card computing power cluster can achieve a continuous training effective time of more than 99%, and the model computing power utilization rate can be increased by more than 20%, supporting a single cluster ten-thousand-card level AI computing power scale.
After improving the training efficiency of large models, it is also necessary to continuously improve the inference efficiency of large models, which will directly affect the cost of enterprises using large models.
The development of large models in the past two years has followed the Scaling Law (a law proposed by OpenAI in 2020, directly translated as "scaling law") - the performance of the model is mainly related to the size of the amount of calculation, the number of model parameters, and the amount of training data.
A core person in charge of the cloud vendor's large model business mentioned that the core principle of cloud vendors is to improve data quality and quantity, appropriately reduce model parameters, and improve model performance under the constraints of the Scaling Law; it is also possible to use the MoE (Mixture of Experts, a model design strategy, by mixing multiple specialized models, to obtain better performance) architecture to improve model performance and reduce inference costs.
In terms of specific business strategies, there are two plans.
First, by increasing data quality/quantity, optimizing algorithms and architecture to improve model performance and reduce model size.
This can effectively reduce the consumption of computing power, improve the main application effects, and adapt to the mainstream market demand.
Second, adopt a more accurate and segmented model product strategy.
Do not expect to solve all problems with a few models, but let different models solve different problems.
For example, let the cost-effective model cut the economic market, and let the high-quality model cut the high-end market.
The computing power structure of cloud computing is undergoing dramatic changes.
Now consuming more inference computing power means that it will grab more incremental markets.
Alibaba Cloud has been leading in the CPU-based computing power stage, and it needs to ensure its advantage in the GPU-based computing power stage.International market research firm IDC forecasts that from 2022 to 2027, China's general computing power will have a compound annual growth rate (CAGR) of 16.6%, while intelligent computing power will have a CAGR of 33.9%.
Within intelligent computing power from 2022 to 2027, the proportion of inference computing power will rise to 72.6%, while the proportion of training computing power will decline to 27.4%.
In May of this year, Chinese cloud service providers initiated a price war for large model inference computing power.
ByteDance's cloud service Volcano Engine, Alibaba Cloud, Baidu Intelligent Cloud, and Tencent Cloud successively reduced the prices of large model inference computing power by more than 90%.
Recently, several cloud service technology professionals have indicated to us that before May, the gross margin of domestic large model inference computing power was higher than 60%, which is basically consistent with international counterparts.
After the consecutive price reductions in May, the gross margin of inference computing power has decreased significantly.
A senior executive of a leading cloud service provider said in June this year that he had conducted multiple rounds of internal deliberation and calculation on the logic of price reduction, identifying two contradictory points.
After the price reduction, the existing revenue will decrease, but the incremental revenue will increase.
Ideally, the incremental revenue should be able to cover the existing revenue.
Second, if peers reduce prices more aggressively, how should they respond?
The ultimate conclusion is that current scale is more important than profit, and short-term revenue can be sacrificed for expected long-term growth.
In fact, the decline in the price of large model inference is meaningful for the AI large model industry, which is still in its early stages of development.
In the short term, the revenue that inference computing power can bring is not much.
A Chinese cloud service technology professional explained that the revenue from model calls by various companies in 2024 will not exceed 10 billion yuan, which is limited in the scale of hundreds of billions of yuan in annual revenue.
However, in the next 1-2 years, the number of large model calls is expected to grow exponentially by more than 10 times.
If the number of calls is large enough, long-term revenue growth will be able to offset short-term revenue losses.
According to the law of technological development, during this process, AI applications will gradually increase, and computing power costs will gradually be spread out with the growth of customer demand.
The large model business still has the opportunity to achieve positive profits, and it is very likely to become a new growth point for cloud service providers.
Before September this year, the price of large models of Chinese cloud service providers was only 20%-50% compared with the same specifications of AI startups like OpenAI.
Taking Alibaba's Tongyi Qianwen-Max, Baidu's ERNIE-4.0-8K, and Tencent's hunyuan-pro as examples of three flagship models, the output price per million tokens of the three models is 120 yuan, 120 yuan, and 100 yuan, respectively.
Their counterpart, OpenAI's flagship model GPT-4-turbo, has an output price of 210 yuan per million tokens (OpenAI's official price is $30, which has been converted to RMB at an exchange rate of 1:7).
The price of these three domestic large models is only about 50% of GPT-4-turbo.
Over the past year, the API (Application Programming Interface, like a switch for water and electricity, consuming tokens when called) calling output price of Alibaba's Tongyi Qianwen large model has decreased by 97%, and the entry-level model's million tokens calling price has been reduced to 0.5 yuan.
Another consideration for Alibaba Cloud is that large models can also increase the penetration rate of cloud computing in the entire industry - the price reduction is a win-win strategy for the industry and itself.
Information disclosed by Alibaba Cloud shows that after the first round of price reduction, a large number of enterprise users called the Tongyi large model, and the number of paying customers on Alibaba Cloud's Bailian platform increased by more than 200% compared to the previous quarter.
At present, Alibaba Cloud's attitude towards lowering the price of large models is firm.
On September 19th at the Yunqi Conference, Zhou Jingren announced the price reduction of Tongyi's three main models again.
Data released by Alibaba Cloud shows that the input price of Tongyi Qianwen-Max has been reduced by 50%, and the output price has been reduced by 50%.
The input price of Tongyi Qianwen-Plus has been reduced by 85%, and the output price has been reduced by 90%.
The input price of Tongyi Qianwen-Turbo has been reduced by 85%, and the output price has been reduced by 90%.
Where is the bottom line for the price reduction of large model inference?
A digital enterprise executive believes that this may have to wait until the "killer" AI application truly takes off.
Zhou Jingren's view is that the current large model application innovation is still in its early stages.
If the model usage price is relatively expensive, it will prevent AI applications from being widely implemented.
Alibaba Cloud's decision to reduce the price of each model is a serious assessment and the result of market feedback.
Alibaba Cloud will continue to innovate in technology to reduce computing power costs and pass the benefits to corporate customers.
Zhang Qi, vice president of Alibaba Cloud, believes that the price reduction of large model inference should not be understood with the competitive thinking of "price war".
The model price reduction is like the acceleration and price reduction of telecom operators.
Today's mobile traffic fees are incomparable to those of 20 years ago.
The acceleration and price reduction of telecom operators have given birth to the innovation of the mobile Internet.
Alibaba Cloud is considering a longer-term issue - promoting AI application innovation.
The decline in the price of large model inference will also lead to an explosion of AI applications.
The explosion of AI applications can already be seen in Silicon Valley.
A Chinese cloud service executive mentioned in May this year that at the beginning of the year, he found that the trend of AI application entrepreneurship in Silicon Valley was similar to the trend of China's mobile Internet in the early stages of 2012-2014.
"AI application entrepreneurship teams quickly achieved revenue and financing.
The Chinese market may show this trend in the future.
But the premise is that the price of large model inference is low enough, and the threshold for trial and error is low enough."
Greater computing power expenditure, lower model prices, and higher technical thresholds.
This means that the elimination of large models has already begun - on the other hand, the AI application ecosystem is also sprouting.
Large models need continuous investment, and they need the ability to have ten thousand or even a hundred thousand cards, and they also need commercial returns.
In the view of a Chinese cloud service strategy person, many companies do not have such capabilities.
In the future, there will only be three to five basic model manufacturers in the Chinese market.
The market will gradually clear out, leaving only truly competitive companies.
Wu Yongming mentioned at the Yunqi Conference that the investment threshold for global advanced model competition will reach the level of billions or tens of billions of dollars.
A Chinese cloud service technology person said in September this year that Chinese cloud service providers need to maintain an annual computing power capital expenditure of tens of billions of yuan.
According to the current inference computing power usage, several leading cloud service providers participating in the price war will have to subsidize more than one billion yuan for large model inference computing power in 2024.
Many industry people have expressed the same view to us, this round of elimination will last for one or two years, and only three to five basic model companies can continue to survive.
A technology company's strategic planning person believes that Alibaba Cloud is relatively calm in this elimination.
First, Alibaba Cloud has achieved profitability (profitability under non-U.S. GAAP standards, excluding non-cash factors such as server depreciation and employee equity incentives).
Alibaba Cloud mainly comes from the four major public clouds (computation, storage, network, database), and low-priced models will promote customer business data consumption, thereby driving the sales of the above basic cloud products.
In the long run, the ideal situation for the development of large models is to ultimately rely on high-performance models and reasonable prices to establish a healthy and sustainable commercial loop.
A core person in charge of the large model business of a cloud service provider believes that this logic can only be established after the elimination ends.
At least in the next 1-2 years, the primary goal of many large model manufacturers is to survive this round of price wars.
Despite the increasingly fierce competition of advanced models, a more optimistic judgment is that the continuous reduction of computing power costs and model prices will imperceptibly allow the large model application ecosystem to gradually explode.
As model prices continue to fall, the AI application ecosystem will gradually prosper.
The remaining large model manufacturers will ultimately become the final beneficiaries.
Zhou Jingren said to us that Alibaba Cloud's goal of promoting the prosperity of the large model ecosystem has not changed.
In the future, it will insist on releasing technological dividends to corporate users and developers, and promote the development of the entire AI industry.